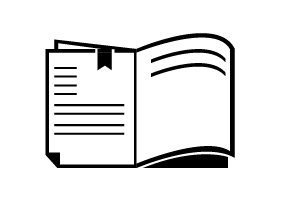
Publication Type:
Authors:
Source:
SPIE Defense, Security & Sensing, Orlando, Florida (2010)Abstract:
Active learning methods have gained popularity to reduce human eort in annotating examples in order to train a classier. When faced with large amounts of data, the active learning algorithm automatically selects appropriate data samples that are most relevant to train the classier. Typical active learning approaches select one data instance (one face image, for example) in one iteration of the algorithm, and the classier is trained with the selected data instances, one-by-one. Instead, there have been very recent eorts in active learning to select a batch of examples for labeling at each instant rather than selecting a single example and updating the hypothesis. In this work, a novel batch mode active learning scheme based on numerical optimization of an appropriate function has been applied to the biometric recognition problem. In problems such as face recognition, real-world data is often generated in batches, such as frames of video in a capture session. In such scenarios, selecting the most appropriate data instances from these batches (which usually have a high redundancy) to train a classier is a signicant challenge. In this work, the instance selection is formulated as a mathematical optimization problem and the framework is extended to handle learning from multiple sources of information. The results obtained on the widely used NIST Multiple Biometric Grand Challenge (MBGC) and VidTIMIT biometric datasets corroborate the potential of this method in being used for real-world biometric recognition problems, when there are large amounts of data.
Authors
Projects
The rapid escalation of technology and the widespread emergence of modern technological equipments have resulted in the generation of large quantities of digital data. This has expanded the possibilities of solving real world problems using computational learning…