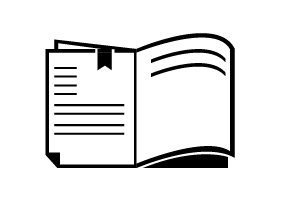
Publication Type:
Authors:
Source:
Workshop on Optimization for Machine Learning at Neural Information Processing Systems (NIPS) (2010)Abstract:
Active learning techniques have gained popularity in reducing human effort to annotate data instances for inducing a classifier. When faced with large quantities of unlabeled data, such algorithms automatically select the salient and representative samples for manual annotation. Batch mode active learning schemes have been recently proposed to select a batch of data instances simultaneously, rather than updating the classifier after every single query. While numerical optimization strategies seem a natural choice to address this problem (by selecting a batch of points to ensure that a given objective criterion is optimized), many of the proposed approaches are based on greedy heuristics. Also, all the existing work on batch mode active learning assume that the batch size is given as an input to the problem. In this work, we propose a novel optimization based strategy to dynamically decide the batch size as well as the specific points to be queried, based on the particular data stream in question. Our results on the widely used VidTIMIT and the MBGC biometric datasets corroborate the efficacy of the framework to adaptively identify the batch size and the particular data points to be selected for manual annotation, in any batch mode active learning application.
Authors
Projects
The rapid escalation of technology and the widespread emergence of modern technological equipments have resulted in the generation of large quantities of digital data. This has expanded the possibilities of solving real world problems using computational learning…