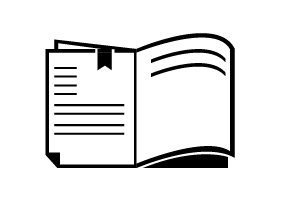
Publication Type:
Authors:
Source:
Computers in Cardiology (CinC 2009), Park City, UT (2009)Abstract:
Drug Eluting Stents (DES) have distinct advantages over other Percutaneous Coronary Intervention procedures, but have been associated with the development of serious complications after the procedure. There is a growing need for understanding the risk of these complications, which has led to the development of statistical risk evaluation models. Conformal Predictors are a recently developed set of machine learning algorithms that allow not just risk classification on new patients, but add valid measures of confidence in predictions for individual patients. In this work, we have applied a novel Support Vector Machine (SVM) based conformal prediction framework to predict the risk of complications following a coronary DES procedure. This predictive model helps to risk stratify a patient for post-DES complications, and the valid measures of confidence can be used by the physician to make an informed, evidence-based decision to manage the patient appropriately.