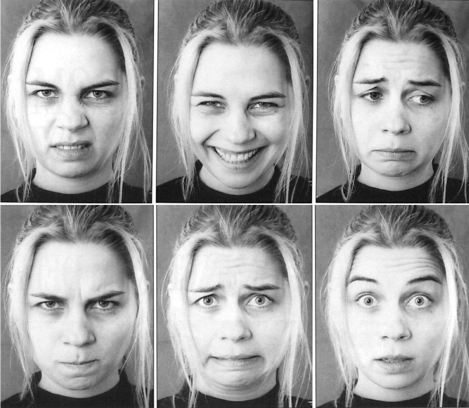
Deep Architectures for Emotion Estimation
Contact
Members
Hemanth Venkateswara
Hemanth Venkateswara
Dr. Sethuraman "Panch" Panchanathan
Dr. Sethuraman "Panch" Panchanathan
Motivated by the vision of the future, automated analysis of nonverbal behavior, and especially of facial behavior, has attracted increasing attention in Computer Vision, Pattern Recognition, and Human-Computer interaction. With facial expression being one of the most effective ways of implicit communication, analysis of facial expression is the essence of most affective computing technologies. Commonly used facial expression descriptors in affective computing approaches are the six basic emotions viz. fear, sadness, happiness, anger, disgust, surprise; proposed by Ekman and discrete emotion theorists. These are a common set of emotions that are universally displayed and recognized from facial expressions.
Most of the facial expression analyzers are directed towards extracting 2D spatiotemporal facial features. The extracted features are usually either geometric features such as the shapes of facial components (eyes, mouth, etc.) and the location of facial fiducial points (corners of mouth, eyes, etc.) or appearance features representing the texture of facial skin including wrinkles, bulges and furrows.
The goal of this project is to apply Deep Learning architectures to the problem of Facial Expression Recognition. Learning features with deep architectures is a relevant choice because highly nonlinear functions can be represented much more accurately with deep architectures than with shallow ones. A Deep Belief Network(DBN) is an example of a deep neural network architecture.